Predictors for extubation failure in COVID‑19 patients using a machine learning approach
Introduction
Extubation(발관)을 시행할 최적의 타이밍을 결정하는 것은 중환자의학 분야에서 주요한 주제 중 하나이다. 본 연구에서는 COVID 19 중환자에서의 extubation failure를 예측하는데 어떤 인자가 있는지 확인해 보았다.
Methods
다기관에 등록된 3,464명의 COVID 19 성인 중환자를 대상으로 하였으며, demographics, clinical observations, medications, fluid balance, laboratory values, vital signs, life support devices에 대한 data를 확인하였다. 적어도 한 번은 extubation을 시도하였던 모든 환자를 분석 대상으로 하였다. 타병원에서 전원 된 환자, 24시간 이내에 중환자실에서 퇴실한 환자, data extraction 시기에 입원해 있던 환자는 연구에서 제외하였다. Potential predictor는 중환자실 담당의가 결정하였다. Primary outcome은 extuabtion 후 7일 이내 reintubation을 하지 않은 경우, secondary outcome은 extubation 후 48시간 이내에 reintubation을 하지 않은 경우로 하였다.
Fivefold nested cross-validation을 이용하여 여러 machine learning algorithm을 training 및 validation 하였다.
Shapley additive explanation으로 predictor importance를 계산하고, extubation failure의 relative probability에 대한 cutoff value는 partial dependence plots를 이용하여 계산하였다.
Results
총 883명의 환자가 model deivatio에 포함되었다. Reintubation rate은 48시간 이내에 13.4%, 7일 이내에 18.9%였다. Gradient-boost model이 가장 좋은 performance를 보여(area under the curve of 0.70) predictor importance를 계산에 선택하였다.
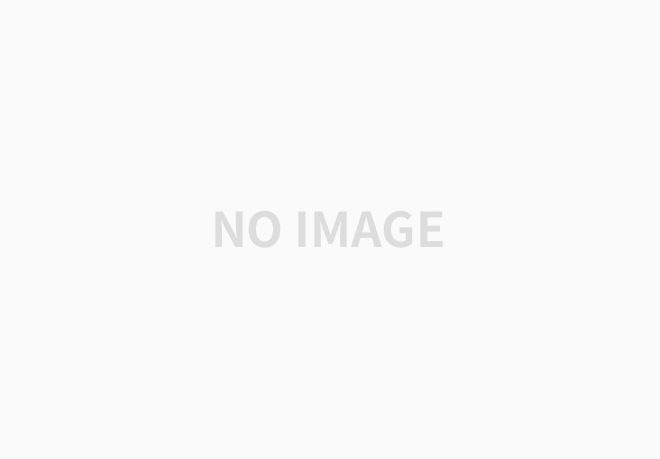
Ventilator characteristcs와 setting이 가장 중요한 predictor로 확인되었다.
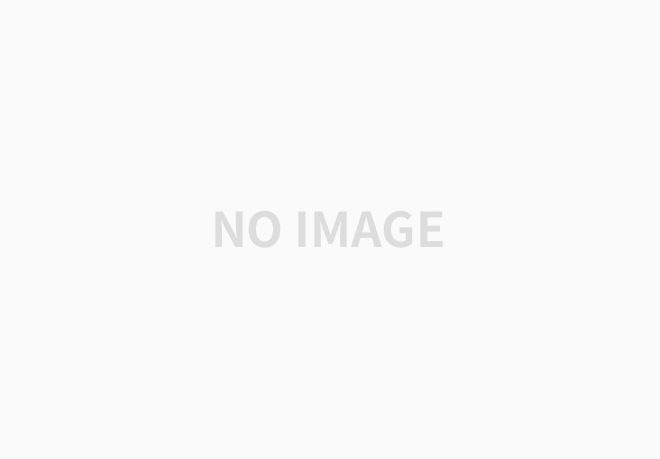
특히 4일 이상 controlled mode를 사용한 경우, 최소한의 inspired oxygen이 45% 이상인 경우, extubation 전 mean tidal volume이 8 ml/kg 이상인 경우, median value와 비교해서 assited mode가 2일 이내로 짧았던 경우가 해당되었다. 또한 median value와 비교해서 C-reactive protein과 leukocyte count가 높았던 경우, thrombocyte count가 낮았던 경우, Glasgow coma scale이 낮았던 경우, body mass index가 낮았던 경우가 extubation failure와 연관이 있었다.
Conclusion
COVID-19 중환자에서 extubation failure를 가장 잘 예측하는 인자로 ventilator settings, inflammatory parameters, neurological status, body mass index를 들 수 있다. 따라서 이와 같은 인자들은 electronic health record에 routine 하게 기록해야 한다.
TEXT
본 연구는 Transparen reporting of a multiavariable prediction model for individual prognosis or diagnosis(TRIPOD) guideline에 따라 진행하였다
Collins GS et al. Transparent reporting of a multivariable prediction model for individual prognosis or diagnosis(TRIPOD): the TRIPOD statement. BMJ. 2015;350:g7594.
본 연구에서는 Dutch Data Warehouse(DDW)를 이용하였다. 총 25개 병원의 데이터로 nested cross valdiation을 하여 model performance를 평가하였다. 데이터를 5 outer fold로 나눈 후 train set와 test set로 배정하고, 각 train set는 다시 5 inner fold로 나누었다. Inner fold를 randomized hyperparameter search로 training 한 후 outer fold test set로 model performance를 test 하였다. 한 환자의 observation은 동일한 split에 배정함으로써 정보의 leakage를 방지했다. 모든 outer fold test set performance의 평균으로 overal model performance를 계산하였다.
Logistic regression model, decision trees, XGBoost algorhithm으로 training 하여 predictor importance를 확인하였고, area under the receiver operating characteristic (AUROC), Brier score, average precision, calibration loss로 model performance를 비교하였다. Outer fold에 따라서 각각 data imputation, standardization, automated feature selection을 시행하였다. Missing data는 median value로 가늠하였고, predictor는 평균이 0, 표준 편차가 1이 되도록 standarization 하였다.
Automatic feature selection을 위해 Lasso regression을 시행하고, 다른 hyperparameter들을 optimization 하기 위해 L1 regularization을 하였다.
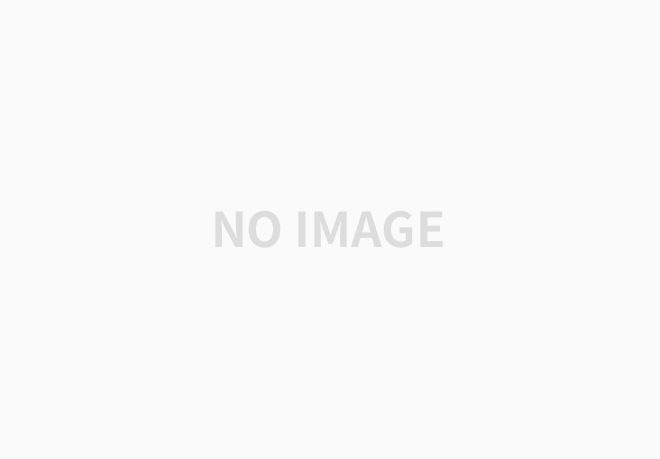
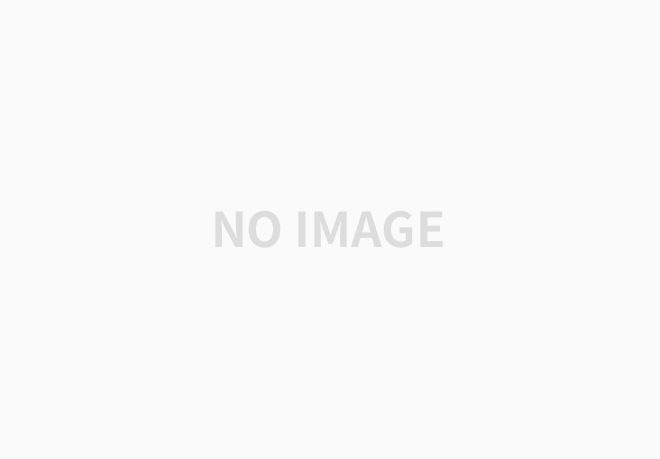
본 연구 결과 BMI가 유용한 predictor로 확인되었지만, 이는 unmeasured predictor 혹은 selsection bias일 가능성이 있다.
이 결과를 실제 임상에서 그대로 참고하기는 어려운 점들이 있다. 환자 치료와 진단 방법을 고민할 때 이용 가능한 parameter들의 variation이 있을 수 있고, 상기 인자들을 extubation 24시간 전에 확인이 가능하지 않는 경우가 있을 수 있다. 예를 들어 NT-pro-BNP나 troponin과 같은 cardiac marker의 경우 routine 하게 검사하지는 않으므로 extubation 실패를 예측할 때 도움이 되지 않을 수도 있다.
Reference
Lucas M. Fleuren et al. Predictors for extubation failure in COVID‑19 patients using a machine learning approach.
Critical Care (2021) 25:448.
2022.05.21 - [의학] - Machine learning을 이용한 패혈증 환자의 Simple Weaning Model
Machine learning을 이용한 패혈증 환자의 Simple Weaning Model
A Simple Weaning Model Based on Interpretable Machine Learning Algorithm for Patients With Sepsis: A Research of MIMIC-IV and eICU Databases Background Invasive mechanical ventilation(침습점 기계 환기)는 sepsis(패혈증) 환자의 예후에서 중
blueorbit.tistory.com
2024.01.13 - [의학] - 중환자에서 SBT(spontaneous breathing trial) 시 T-piece와 PSV의 비교
중환자에서 SBT(spontaneous breathing trial) 시 T-piece와 PSV의 비교
Comparison of T-piece and Pressure Support Ventilation as Spontaneous Breathing Trials in Critically Ill Patients: A Systematic Review and Meta-Analysis Background Extubation 성공과 임상적으로 중요한 다른 결과들에 spontaneous breathing tri
blueorbit.tistory.com
2024.02.06 - [의학] - Theophyllin 투여가 기계 환기 유발 횡격막 기능부전에 미치는 영향
Theophyllin 투여가 기계 환기 유발 횡격막 기능부전에 미치는 영향
Effect of theophylline on ventilator-induced diaphragmatic dysfunction Purpose 본 연구는 ventilator-induced diaphragmatic dysfunction (VIDD)가 발생한 환자에서 theophylline의 투여가 어떤 효과가 있는지 알아보기 위해 진행하였
blueorbit.tistory.com
2024.03.04 - [의학] - COVID-19 환자에서 tocilizumab(악템라주) 투여 효과
COVID-19 환자에서 tocilizumab(악템라주) 투여 효과
Tocilizumab in Patients Admitted to Hospital with COVID-19 (RECOVERY): A Randomized, Controlled, Open-label, Platform Trial Background 이 연구는 COVID-19로 입원 중인 성인 환자에서 hypoxia와 systemic inflammation을 보이는 상황에서 to
blueorbit.tistory.com
2024.03.05 - [의학] - COVID 19 환자에서 D-dimer 상승의 의미
COVID 19 환자에서 D-dimer 상승의 의미
D-dimer and Death in Critically Ill Patients with Coronavirus Disease 2019 Objectives COVID 19(coronavirus disease 2019) 환자에서 나타나는 hypercoagulability(응고 항진성)는 급성 장기 손상과 사망의 주요 기전으로 알려져 있
blueorbit.tistory.com
'의학' 카테고리의 다른 글
Lactate은 외상 환자의 병원전 단계에서 plasma 수혈의 효과를 보여준다 (0) | 2022.06.19 |
---|---|
Sepsis-induced DIC 환자에서 antithrombin을 언제 투여해야 할까? (0) | 2022.06.08 |
Machine learning을 이용한 패혈증 환자의 Simple Weaning Model (0) | 2022.05.21 |
NOACs을 복용 중이던 환자가 응급 수술을 받는 경우 (0) | 2021.11.15 |
수술을 앞둔 환자에서 NOACs를 언제 중단해야 할까? (0) | 2021.11.15 |